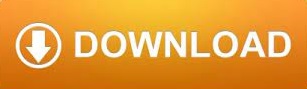
This allows us to understand a tradeoff between following schedule and seeking profits, and thus, evaluate how profitable it may be to deviate from schedule. We also consider an alternative model to incorporate a benchmark trading schedule and show that our model is capable of generating optimal strategies that very closely follow any given schedule while minimizing trading cost. Among our results, we characterize the trader’s buy-sell boundary, which is a time deterministic function governing when order types are non-negative.

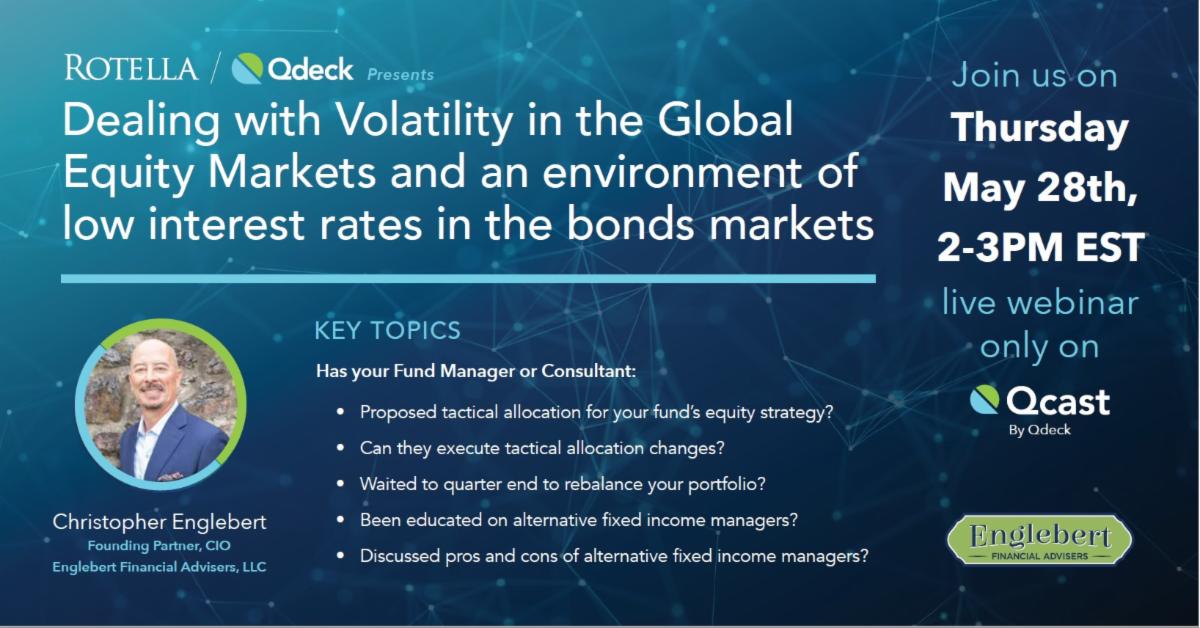
We then explore the special cases of constant and linear uncertainty to examine the properties of the solutions and analyze the corresponding explicit optimal strategies. We model this fill uncertainty using an affine function of the trading rate in limit orders this leads to an additional diffusion term that is correlated with the stock price process. The optimal liquidation problem is then cast as a stochastic optimal control problem, whose associated nonlinear Hamilton-Jacobi-Bellman (HJB) equation can be simplified to a system of linear ordinary differential equations (ODEs). The aggressive nature of market orders tends to result in a higher market impact, whereas limit orders have less market impact but are less likely to fill.
Qcast podcasts and how to#
Our objective is to investigate how to optimally allocate aggressive (market) and passive (limit) orders over time to achieve trading goals. Finally, our model is capable of generating optimal strategies that very closely follow any given benchmark trading schedule while minimizing trading cost.
Qcast podcasts and full#
In combination, these three penalties ( non-liquidation penalty, trade director, and speed limiter) force the algorithm to trade to full liquidation, while simultaneously tailoring the sign and magnitude of the trading rates. Specifically, we include a non-liquidation terminal penalty as well as a penalty, called trader director, to push trading rates for market and limit orders to be in the same direction. submission of sell orders in a buy program or vice versa).Īdditionally, we construct penalties that drive the optimal trading rates in the desired direction of complete liquidation. Unlike Almgren & Chriss (2000), our model has two order types, which can potentially have negative signs (i.e. Our article extends the foundational market impact model of Almgren & Chriss (2000) by including limit orders with uncertain fill rates, and a speed limiter that penalizes overly large trading rates. In our paper, we analyze a continuous-time stochastic model for optimal execution using both market and limit orders. Hence, it is the goal of many algorithmic traders to decide how to strategically trade off cost and risk for an institutional trade. However, this strategy exposes the agency trader to the risk in the stock price movement as well as the failure to complete the buying/selling of all desired units of stocks. This can be done by slowly trading the asset, which usually reduces market impact by minimally affecting demand/supply over time.
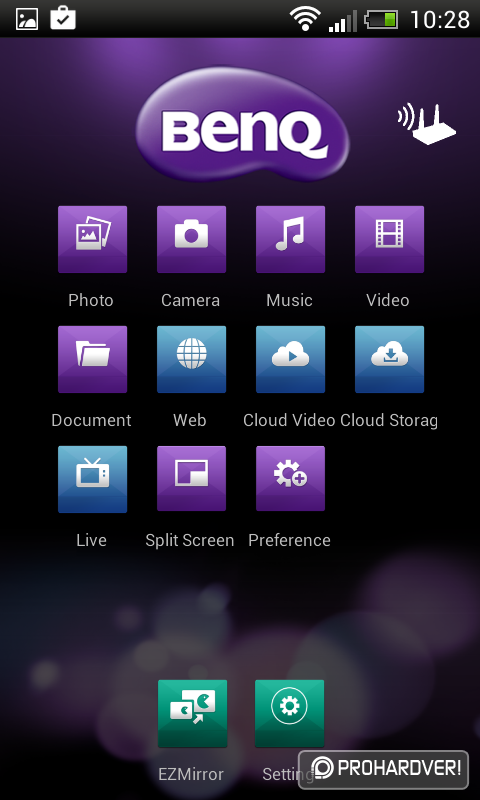
Practitioners commonly view this as a significant cost to track, avoid or minimize. This additional implementation cost due to short-term liquidity demand is often called market impact. Such orders are thought to be potentially costly to implement, as they create substantial immediate demand/supply and possible adverse price effects.Ī large buy/sell order may cause other traders to raise/lower their offered price because they perceive a change in value from the contra orders of the institutional investor. For many institutional investors, orders to buy or sell stocks can be very large. In the US market, institutional investors own or manage a major share of public equities.
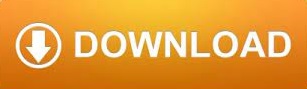